In healthcare tendering, game theory principles are often applied to predict the winning price at a tendering opportunity.
Game theory is the study of strategic interaction between participants. A player’s decision affects the actions of the other players and vice versa. Players in game-theory can be people, companies, countries, or even machines. At our “healthcare tendering games,” players are competitors bidding for the healthcare tenders’ winning price.
Competitors at tendering opportunities make decisions. The outcome, however, often depends on the decisions that other people make.
Game theory, the most influential model of choice in economics and the social sciences, offers an answer, based on games of strategy such as chess and checkers: the entity considers the choices others will make and makes a decision that will lead to a better result.
The game of price-tendering in Pharma
In a price-based tender it is extremely common for the lowest price to bring home the bacon. As such, tendering is regularly deployed for healthcare goods that are produced by multiple manufacturers in order to acquire the product with the lowest price.
Whenever we have a situation with two or more players that involve known payouts, game theory can be used to help determine the most likely outcomes. This is the case with price-based tendering in the pharmaceutical industry
Each competitor’s revenue depends on its bidding price and the price bid by rival providers. Hence, providers face strategic interaction. The payoff each company gets from its chosen price will depend on its rivals’ choice.
Players are unaware of the other players’ actions when making their own decisions. Games with this characteristic are called “simultaneous move games.”
How real-world refutes game theory: The fallacy of the rational choice
In our practice at price-based tendering, we rarely gather evidence that suggests human decision-making is not governed by rationality. Nevertheless, bidders quite often play against their own benefit.
Each tendering space is determined by different conditions that alter human decision making. Therefore, one predictive analytics solution will not fit all. Empirical data refutes nicely woven theories time and time again.
The idea of human rationality is incredibly old. From a neoclassical economics standpoint, early instances of human rationality can be found in the notion of people being “homo economicus”; that is, rational decision-makers, who choose logically, based on the information they have and who seek to maximize their benefit.
Unfortunately, at the very best, we could talk about limited rationality. Human rationality is limited by the manageability of the decision problem; namely, how easy it is to handle the cognitive limitations of our mind, the time available to make the decision, and how important it is to us.
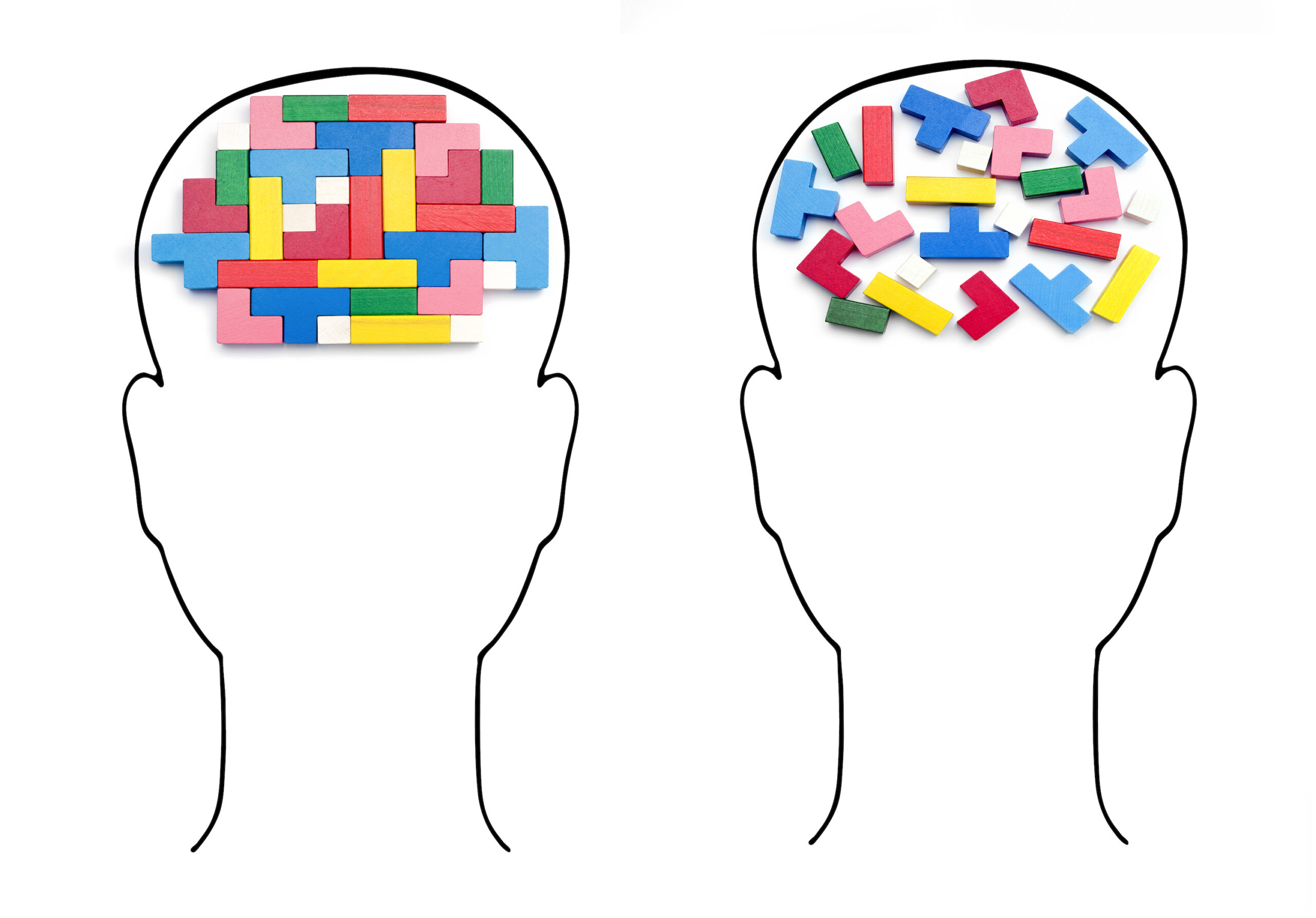
How do algorithms model limited rationality in decision making
We often make decisions that are anything but rational, and we are imbued with beliefs that have little to do with reason and data.
The game theory presents a set of axioms that players must “satisfy” to be considered “rational.” This generates “rigorous” and “precise” conclusions, but they rarely correspond to what happens in the real world.
This line of reasoning helps us model algorithms that anticipate other players’ biddings.
But any model that succeeds in real-life tendering should be informed by empirical data based on real observations, which, in real life tendering, contradict the axioms of game theory
As a result, while aware of game theory components, successful models should be ready to react to behaviors that play against mutual benefit.
CONTACT US TO SCHEDULE A SHORT MEETING!
HEALTHCARE TENDERING AT KONPLIK
Konplik has over six years of experience in market intelligence for tendering in healthcare. Within the area of tender management, we have been actively delivering hundreds of projects to leading healthcare companies in more than 40 countries.
These are our areas of expertise:
1) Scraping: We gather unstructured information, which, when working on the WWW, is dealt with using scraping.
2) NLP structuring: We convert the raw source into a structured or semi-structured format.
3) Transformations: We combine the data we gather with the company’s private data.
We, then, perform complex and custom transformations – including custom filtering, fuzzy product matching, and fuzzy de-duplication on large sets of data.
4) Business ready insights: Subsequently, we apply any standard predictive analytics or data mining techniques to extract insights.
5) Prediction: We also use Artificial Intelligence-based algorithms to predict and optimize revenue and margins, as well as to expand the discovery of new business opportunities.
Recent Comments