Hybrid approaches that blend experts’ forecasts with complex machine learning algorithms are often the best tool for accurate healthcare demand forecasts.
Healthcare demand forecasters tend to rely heavily on judgment, despite the inherent bias and inefficiencies associated with it. We find that those sub-optimal forecasting techniques often compromise forecast accuracy.
According to the Lancaster Centre For Forecasting
- In the pharmaceutical industry, demand forecasting is a relatively new task, which helps to explain why most companies (82.1%) rely on simple methods such as:
- Moving averages for the preprocessing of time series,
- The naïve technique in which the last period’s actuals are used as this period’s forecast, without adjusting them or attempting to establish causal factors.
- The Delphi Technique, which relies on the experts’ panel. See AI for demand forecast in healthcare.
- Demand Forecasters tend to rely heavily on judgment, despite the inherent bias and inefficiencies associated with it.
- Spreadsheet Software in Forecasting is the most commonly used type of software.
- The average one-month and three-month ahead item level MAPE is around 40%.
REFERENCE
Weller, M., and S. Crone. (2012) “Supply Chain Forecasting: Best Practices & Benchmarking Study.” Lancaster Centre for Forecasting, 41. https://core.ac.uk/download/pdf/224768371.pdf
Machine learning for demand forecasting
Nowadays, contemporary methods rely on artificial intelligence algorithms that “learn” from experience and analyze many complicated relationships and constituents that influence product demand.
Machine learning for demand forecasting has grown to an industry level that can dramatically transform business in Pharma.
For starters, machine learning-based algorithms constantly update data and models to adapt to offer and demand changes. They are also faster than experts’ decisions.
We have developed tailor-made algorithms based on gradient boosting techniques. Gradient boosting is a machine learning technique that we use for regression in demand forecasting. We have discovered that gradient boosted trees outperform random forest in demand forecasts for the Pharma Industry.
Usual metrics and business metrics
As for metrics, to assess the quality of their forecasts, most companies use variations of the following basic metrics:
- Forecast Bias
- Mean Absolute Deviation (MAD)
- Mean Squared Error (MSE)
- Mean Absolute Percentage Error (MAPE)
Although, at Konplik we do not think of demand forecasts as an end goal. Forecasting accuracy is a means to increasing value for the company.
More accurate demand forecasting improves customer service and inventory management. It also reduces backorders and costs incurred due to breach of contract.
We identify and quantify the impact of an improved forecast on the organization – Most importantly, we put numbers on the expected benefits.
Accordingly, our algorithm architecture is modeled to deliver the best business results in demand forecasting, taking into account the product lifecycle, inventory backorders, contract penalties, and customer service.
CONTACT US! TO SCHEDULE A MEETING
DEMAND FORECAST IN HEALTHCARE
Konplik has several years of experience in demand forecasting in healthcare.
1) Tailor-made algorithms. Experts along with AI: Hybrid approaches that blend experts’ forecasts with complex machine learning algorithms are often the best tool for accurate healthcare demand forecasts.
2) Business-oriented forecasting: More accurate demand forecasting improves customer service and inventory management. It also reduces backorders and costs incurred due to breach of contract.
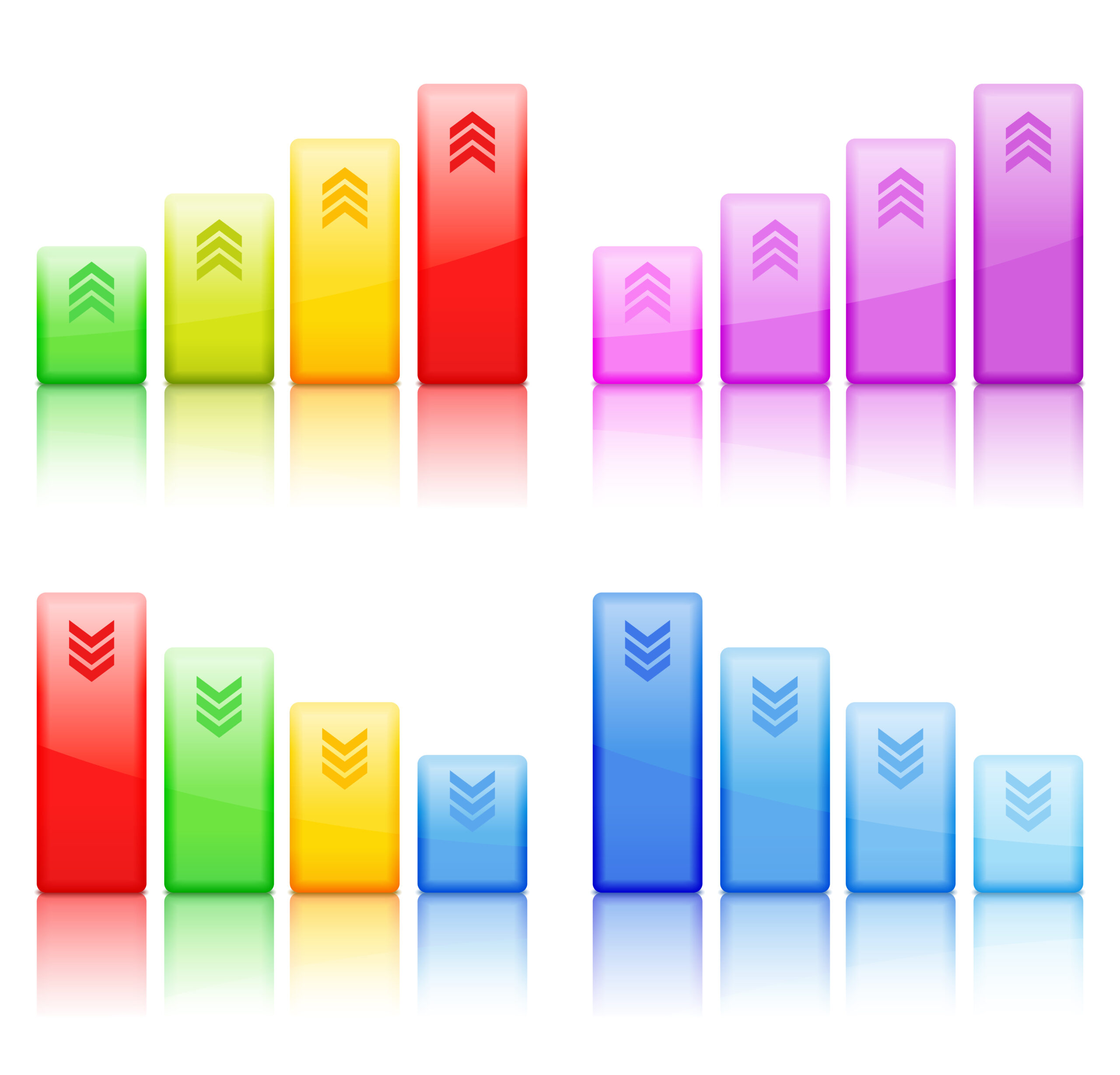
Recent Comments